Understanding Semantic Segmentation in Video: Enhancing Data Annotation Tools
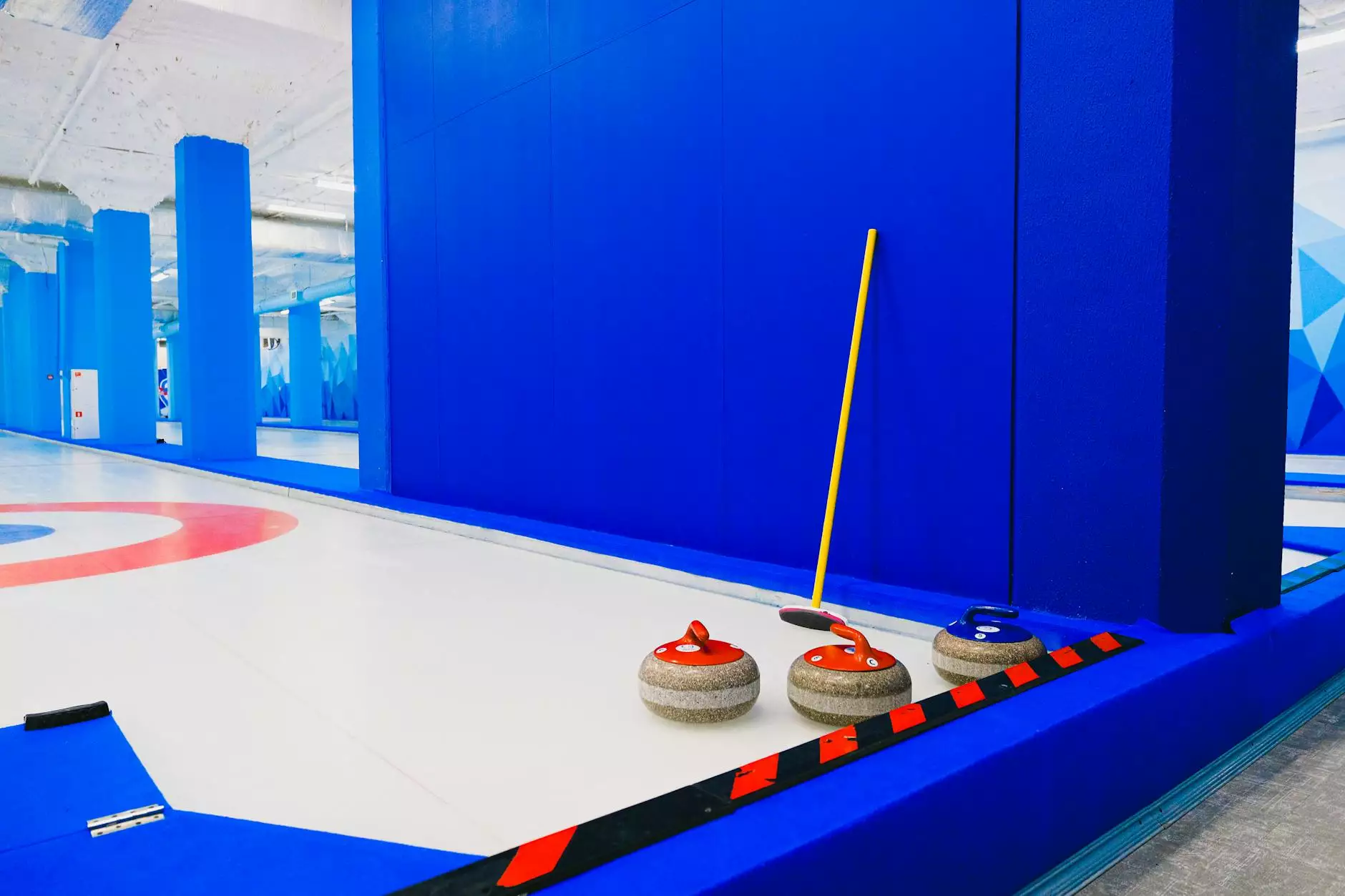
In the contemporary landscape of machine learning and artificial intelligence, the vast amount of visual data generated daily presents both opportunities and challenges. Businesses strive to extract meaningful insights from this data, and one of the most effective methods to achieve this is through semantic segmentation video. In this article, we will explore the mechanisms of semantic segmentation, its significance in the realm of data annotation tools, and how platforms like Keylabs.ai are paving the way for advanced data processing technologies.
What is Semantic Segmentation?
Semantic segmentation is a computer vision task that involves classifying each pixel in an image into predefined categories. This technique is pivotal in understanding scenes within images and videos, allowing computers to perceive and interpret visual information akin to human understanding.
For instance, in a video of a city street, semantic segmentation helps identify and isolate different elements such as roads, buildings, pedestrians, and vehicles. Each pixel of the image is tagged, creating a detailed map of the scene that enhances the machine's ability to understand context.
The Importance of Semantic Segmentation in Video Processing
Semantic segmentation in video formats brings a myriad of benefits, especially in various industries such as autonomous driving, surveillance, and interactive media. Below are a few key advantages:
- Enhanced Object Detection: Semantic segmentation allows for precise localization and differentiation of objects within a frame.
- Improved Scene Understanding: It provides deeper insights into the relationships between different elements in a frame, enhancing interpretative capabilities.
- Data Reduction: By focusing on essential segments of the video data, businesses can reduce redundancy and improve processing speeds.
- Increased Model Accuracy: Models trained with semantically segmented data tend to perform better in real-world applications, as they learn to recognize patterns more effectively.
How Does Semantic Segmentation Work?
The process of semantic segmentation can be broken down into several crucial steps:
- Data Collection: High-quality video data is collected from various sources. For effective training, diverse datasets that represent different scenarios and contexts are essential.
- Data Annotation: This step involves using sophisticated tools to label each pixel of the video frames. Accurate annotations are critical for training machine learning models.
- Model Training: With annotated data, deep learning models such as Convolutional Neural Networks (CNNs) are trained to identify and classify each segment in the video.
- Model Evaluation: Post-training, the models are rigorously tested for accuracy and efficiency. Tweaks and adjustments are made based on performance outcomes.
- Deployment: The final model is deployed for practical applications, ready to analyze new video data in real-time or batch processing scenarios.
Data Annotation Tools: The Backbone of Semantic Segmentation
High-quality data annotation tools are fundamental to the success of semantic segmentation. These platforms enable the effective labeling of vast datasets, ensuring that the machine learning models receive precise information to learn from. Keylabs.ai offers advanced data annotation tools designed for efficiency and scalability.
Features of Keylabs.ai Data Annotation Tool
Keylabs.ai stands out in the market due to its innovative features:
- Intuitive User Interface: A user-friendly design that streamlines the annotation process, making it accessible for both novices and experts.
- Collaboration Tools: Features that enable multiple users to work on the same project, enhancing productivity.
- Automated Annotation: Utilizing AI to speed up the annotation process while maintaining accuracy.
- Quality Control Mechanisms: Built-in QA checks ensure that annotated data meets industry standards.
- Flexible Integration: Ability to integrate seamlessly with existing machine learning workflows and tools.
Applications of Semantic Segmentation in Various Industries
The impact of semantic segmentation can be felt across numerous sectors. Here are some of the notable applications:
1. Autonomous Vehicles
In the realm of autonomous driving, semantic segmentation plays a crucial role in navigating environments safely. By accurately identifying roads, traffic signals, pedestrians, and other vehicles, these models help self-driving cars make informed decisions, significantly enhancing road safety.
2. Healthcare
Medical imaging greatly benefits from semantic segmentation. In radiology, for instance, precise highlighting of areas such as tumors or organs in imaging studies enables practitioners to diagnose diseases with greater accuracy, thereby improving patient outcomes.
3. Retail Industry
In retail, semantic segmentation aids in understanding customer behavior through video analytics. It allows businesses to analyze shopper movements, optimize store layouts, and enhance the overall shopping experience.
4. Environmental Monitoring
Semantic segmentation is utilized to monitor environmental changes through satellite imagery. By classifying different land covers such as forests, water bodies, and urban areas, policymakers can make informed decisions regarding environmental conservation.
Challenges in Semantic Segmentation
Despite its benefits, semantic segmentation does face several challenges:
- Data Quality: High-quality annotated data is imperative; subpar data can lead to poorly trained models.
- Computational Resources: Training models for semantic segmentation can be resource-intensive, requiring significant computational power and time.
- Generalization: Models trained on specific datasets may struggle to generalize when exposed to new environments or scenarios.
The Future of Semantic Segmentation in Video
The future of semantic segmentation video is bright, with advancements in deep learning techniques and improved data annotation tools significantly boosting the capabilities of machine learning models. Innovations in areas such as:
- Transfer Learning: This technique allows models trained on large datasets to be refined for specific tasks with less data, improving efficiency.
- Real-Time Processing: Advances in hardware and algorithms will enable real-time semantic segmentation, opening up new possibilities in fields like augmented reality and interactive environments.
Conclusion: The Significance of Semantic Segmentation
In conclusion, semantic segmentation video is a transformative technique that enhances the capabilities of data annotation tools and platforms. By enabling more precise classification of visual data, it opens doors for innovation across various industries, from autonomous vehicles to healthcare. As businesses like Keylabs.ai continue to develop and refine their data annotation platforms, the impact of semantic segmentation will undoubtedly grow, solidifying its position as a pivotal technology in the data-driven age.
To stay ahead in this competitive landscape, companies must embrace these advancements and leverage the power of semantic segmentation to unlock the true potential of their data.